Targeted Advertising: How it works and where it is failing
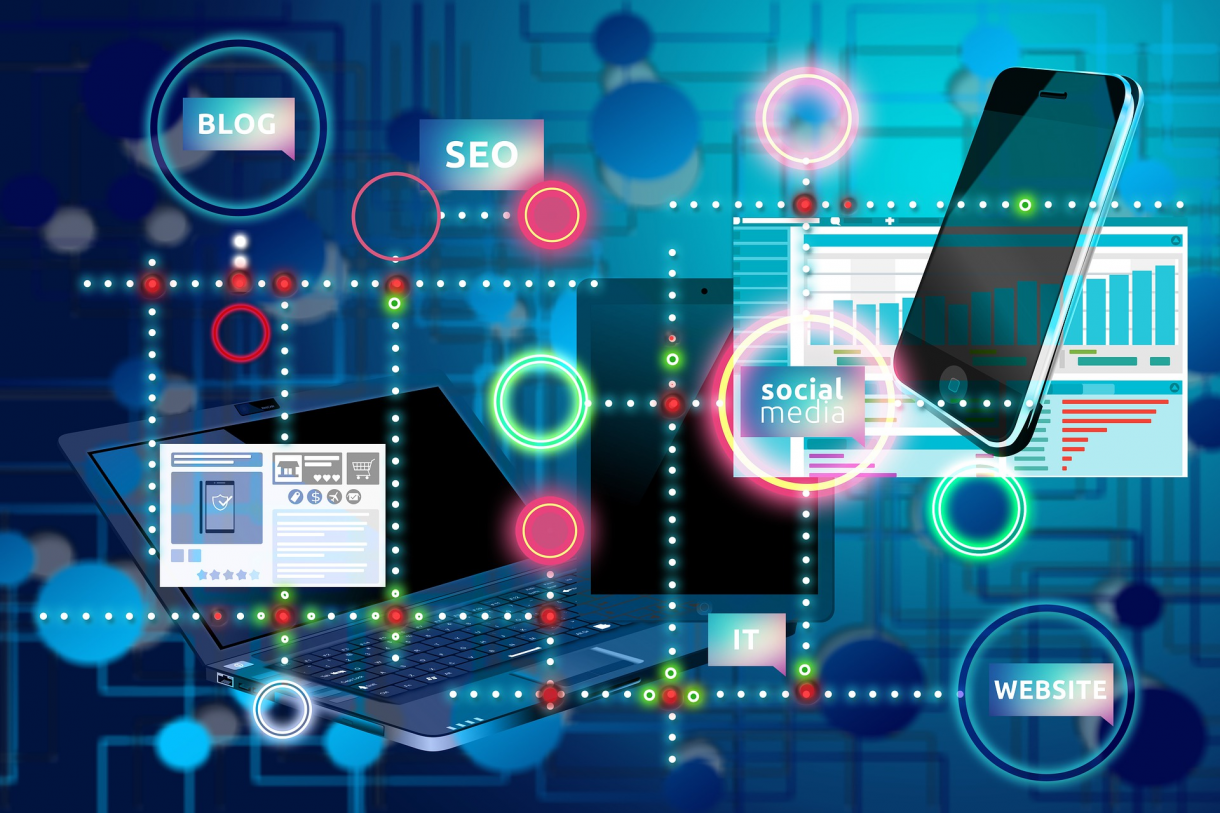
Share this Post
Targeted advertising, also referred to as microtargeting, is a practice wherein a predefined group of users is identified on a platform and selected ads – created by the advertiser at an auctioned price at a certain time – are displayed to them.
This advertising process is at the core of the business model of platforms like YouTube, Twitter or Meta and the profit it generates is still increasing. Since the US election in 2016, followed by the Cambridge Analytica scandal and Brexit, targeted advertising has become the subject of a broadening debate in society and it is discussed more critically than in previous years.
Targeted Advertising – an Introduction
Ads online can take many different shapes and can include various forms of content, depending on what product or platform they appear on. The forms of digital advertising can be divided into the following categories, according to Karolina Iwańska: Behavioral ads and contextual ads, ads displayed on a platform, and advertising that is displayed to the user in the shape of banner ads anywhere on the web. The difference between behavioral and contextual ads depends on whether the ad’s content is tailored to the context, e.g. targeting a beer drinker at a football game or advertising a commuter service on a subway, situations in which the user is likely to encounter or uses patterns within big data about to tailor the ad even more perfectly to the target.
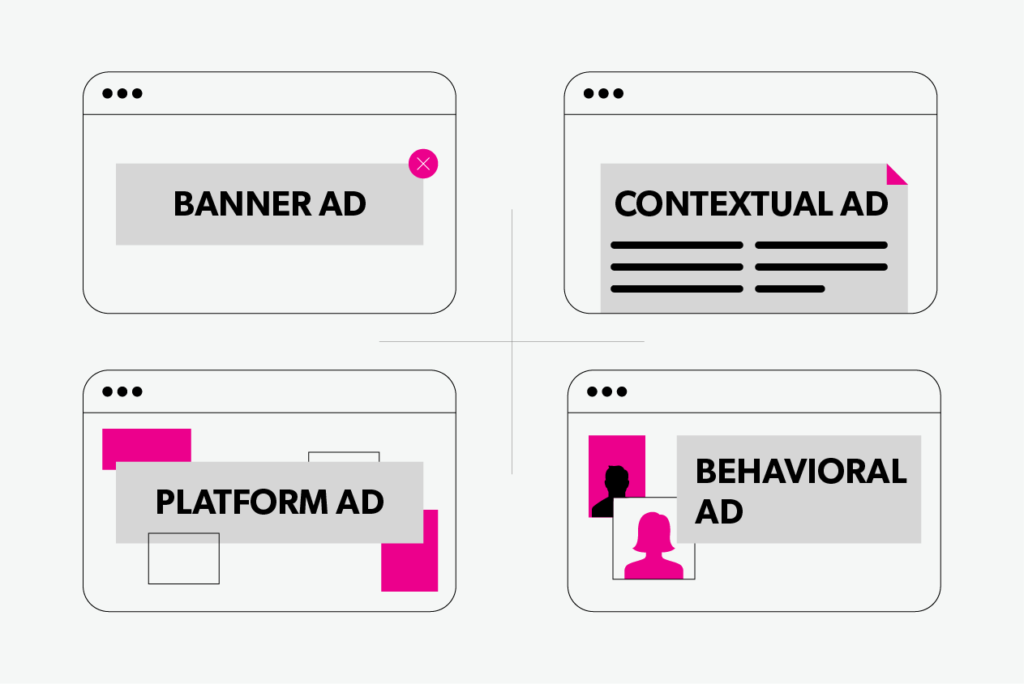
Fig. 1: Illustration distinguishing between banner ads, contextual ads, platform ads and behavioral ads.
The process of targeted advertising involves three stages. In the first stage, the ad is created; in the second, the ad is delivered; and in the third, the ad is displayed.
Targeted ads involve at least three stakeholders throughout this process: the advertiser, the platform, and the user. The first creates the ad, including the selection of visuals and images, text or video content, the decision about what criteria and attributes will determine who should see the ad (“audience selection”) and the decision about the ad’s goals and the price range that advertisers will be willing to pay for to reach a certain group of users. These are later specified in the auctioning of the ad. The platform serves as the intermediary between the advertiser and the user. Through the use of (historic) behavioral data, it defines the audience to which the ad will be displayed at the end of the process.
The setting of the price depends on the audience the advertiser wants to advertise to. The selection therefore is closely related to the attributes the target audience is defined by. The price for political advertising for example can vary accordingly to the attributes ascribed to a certain user, as well as the fit between the message of the advertising itself and the ascribed view and interests ascribed to the target, e.g. the display of a republican political advertisement to a potentially republican user would be cheaper than showing the same ad to a potentially democratic user. The criterion widely used to formulate goals and measure success on big platforms is user engagement. Following this economic strategy, platforms maximize effort to increase the time spent by users on their service or platform in order to display to them the maximum amount of (relevant) ads. The platform also aims for the perfect placement to get the user’s attention, as well as to find the perfect moment in time to display advertising content in the right context to the user. This moment of timing in the ad display process may aim at goals like to find the moment in a user-session in which it is most likely to engage with the content, e.g. to comment on something or to share a post.
Big Platforms
Big platforms, such as Meta have implemented an auction system between the ad-creation and audience-selection phases, to decide on the price per ad. Such auctions of big global operating platforms work with algorithmic support. Meta for example uses the “total value” to decide who won the auction among the different competitors. This “total value” has, according to the company three main factors that determine the success within the bidding competition: the bid itself, the estimated action rates and the quality of the ad. The first is referring to the target the advertiser has. This includes the placement of the ad as well as the advertiser’s budget. The second is referring to the pricing of a prediction that a desired user reaction is performed. The third however is trying to capture the perceived quality of the advertised content itself. While taking into consideration the language used, the degree of information provided or feedback collected through user data. This auction depends on the objective of the ad as well as on the bidding strategy the advertiser has chosen.
On Meta’s platform, advertisers can decide between three different ad objectives:
Awareness, considerations, and conversions. Awareness refers to optimizing an ad for generating as many views as possible. Consideration is a maximizing strategy for user engagement manifesting itself in shares, clicks, or other reactions. Conversion is the goal to optimize the click-through rate, this means the “percentage of times people saw your ad and performed a link click”.
After the target is set, the advertiser has to choose a bidding strategy. In this phase, the advertiser can define bidding caps for the ads, set ranges, define the display duration, and determine the overall campaign budget.
While advertisers define the objectives and bidding strategy of their ads, the platform’s algorithm ultimately decides which user is matched to which of the advertiser’s target groups and how much money the advertiser has to spend to display an ad to its target group within the time frame.
This algorithmic process is very obscure, while also of critical importance within the context of political campaigns. The close look on the user’s (inferred) data, which is used to create a psychometrical understanding of the targeted user supports the platform and the political advertiser with rich information to (hyper-)target the desired group of potential voters. display of ads can influence behavior and nudge a user towards a desired direction and slightly change or support certain behavior e.g. to influence vote choice.
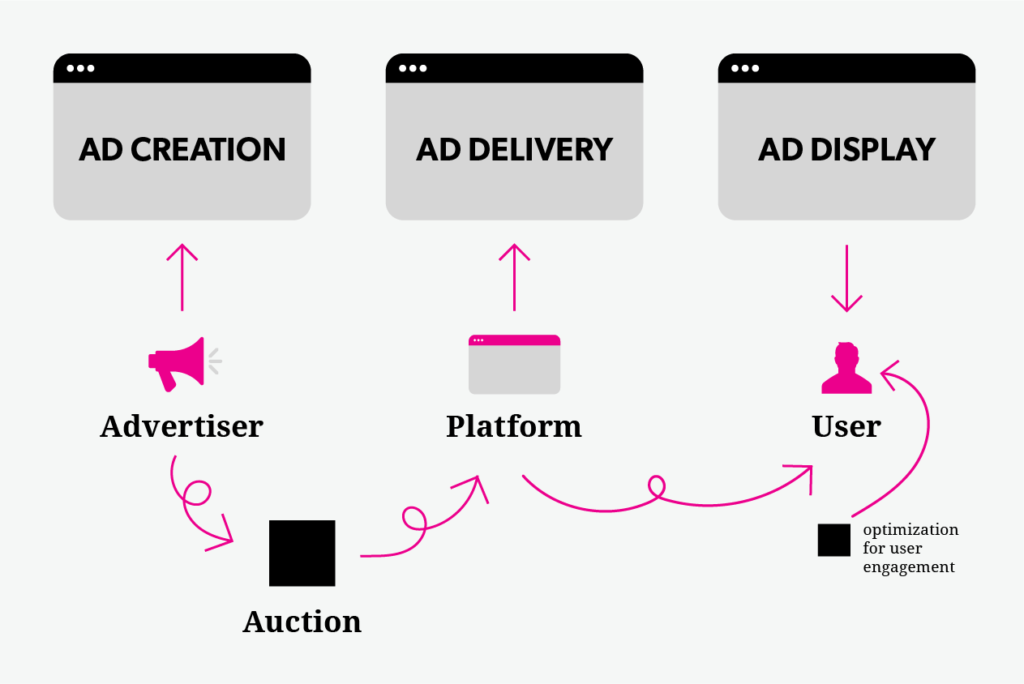
Fig. 2 Advertising phases and respective stakeholders
Problematic Areas of Targeted Advertising Usage
Two manifestations of targeted advertising are especially problematic because they may involve violations in areas (already) protected by law. The first involves the electoral process and electoral campaigns; the second relates to cases of discrimination through targeted ads.
The European Union is actively engaged in the question of how to regulate targeted advertising. European legal frameworks regulating targeted advertising are the eCommerce Directive, the ePrivacy Directive, the Audiovisual Media Services Directive (AVMS), and the P2B Regulation. Furthermore, the Unfair Commercial Practices Directive, the Directive on Misleading and Comparative Advertising, the Consumer Rights Directive, and the General Data Protection Regulation may be relevant in cases concerning targeted advertising. The legislative future of targeted advertising regulation in Europe will also include the Digital Markets Act and the Digital Services Act.
Targeted Advertising in Online Election Campaigns
Online targeting campaigns can very precisely find undecided voters and expose them to tailor-made ads and campaign content at a moment in which they are most likely to interact. This manner of campaigning poses the risk of dark ads or dark posts that only appear to some users and introduce opaque influences and diverging views to political campaigns.
This form of microtargeting voters is seen as endangering the electoral process and threatening to democracy overall. Individuals already at risk of losing faith in the voting process, e.g. users who live in rural areas who are exposed to disinformation about the security of voting by mail, or users interested in conspiracy theories, may become the target of political content such as anti-vaccine propaganda.
This dissemination of various incoherent claims by politicians, conflicting electoral promises and the threat of electoral mis- or disinformation demands for more transparency in digital electoral campaigns. This is including the political parties themselves as well as the platforms that offer their services, as well as advertisers.
Political parties should guarantee that the public has access to the whole corpus of content generated for the entire political campaign including campaigns both – online and offline.
Another very popular way to select an audience is the use of historical user data interpreted by a machine-learning algorithm to generate relevant user or group attributes. Those insights are then used on the data to search for users with similar attributes as the characteristics selected in the previous step. The audience in such a process in the Meta ecosystem is called a Lookalike audience.
When the audience is selected, the mode of display is another important part of the process. One self-regulatory step platforms can take is to support a platform-design-decision in which a warning label is attached to the content category in question (e.g. political content or content labeled as disinformation) to mark an item and to inform the user about who is paying to display the advertising to him or her. In the Meta ecosystem this design choice is known as ‘Why do I see this ad?’ or Twitter’s label for problematic content.
If such a transparency and information supporting feature is implemented on a platform however it must be mentioned that the classification of what counts for political content and therefore should be labeled or not is not entirely clear or easy to classify.
Another design option promoting accountability in the microtargeting process is the ad repository that keeps track of the digital campaigns and the budget used for online election purposes. This ad registry is required of very large platforms (Art 30 DSA) in the draft of the Digital Services Act and demanded by many researchers, academics, and NGOs. Meta has implemented on its platform the Meta ad library. However, there are still currently many problems with this library’s functioning. Among the obstacles are that the repository holds incomplete content, and that it is not user-friendly and easy to understand. Furthermore, both the process between ad creation, ad display, and the (algorithmic) decisions taken during the process are still too opaque and need more research.
Therefore, NGOs and academics have built tools (e.g., browser extensions?) to collect ads and compare them to the content in Meta’s ad library. Who Targets Me – an NGO that investigates political content online – also offered the collected data for download to the public and created a data visualization tool to help people to better understand the presented information in order to increase transparency and inform the public in the recent elections Who Targets Me supported.
Meta furthermore has adjusted the control of ad preferences for users from 2014 on, however not yet in a sufficiently explaining manner.
The Greens party in Germany for its political campaign in the 2021 federal election limited its digital campaign targeting criteria to four attributes only: Age, location, interest, and gender. While this form of self-restriction of a political actor regarding the digital aspects of its campaign is a step forward, it cannot succeed in a vacuum. The campaigning behavior within an electoral process should be aligned between all parties to enable fair and equitable elections, as enshrined in various constitutions or the European Charter. To elaborate on the example of the German federal election, none of the parties followed the recommendations and rules created by Campaign Watch, an electoral observer. Campaign Watch proposed to limit the targeting criteria used to age and location only. An advice the Greens themselves did not follow
In comparison, political campaigning in the offline world is strictly regulated by the different member states. To name some examples from modern democracies: Politicians have to be granted fair presence in public media, disclose their campaign spending, adhere to laws regarding the selection of campaign placement in a city in a manner equitable to all parties, and the implementation of a campaign-free period before the election.
Algorithmic Discrimination
The second problematic sphere at hand is addressing cases of online discrimination through targeted advertising such as the unevenly distributed advertising of open or rare positions in the job marked, high demand rent offers or specific services. Nevertheless, the problem of bias in those cases are no sheer online phenomenon rather, it is a reflection of the inequality and discrimination of the analog world that is manifesting itself in the digital world.
While the amount of online advertising for hiring purposes is on the rise, not only because of its easy-to-use mechanisms, measurements, built-in control tools but also in times of pandemics and reduced traveling globally. However, the potentially unintentional exclusion of marginalized and legally protected groups on the platform may occur for various reasons. Targeted advertising could lead to an increase of anti-discrimination law violations through online behavior or the lack of equal chances to see the same online reality in cases concerning race or ethnic origin or the equality of treatment in employment and occupation within the EU. Prominent targeted advertising examples in the United States were already discussed with regard to Title VII of the Civil Rights Act of 1964 and the Age Discrimination in Employment Act. These legal settings may further open the scope of the advertiser’s liability in recruitment cases.
Discrimination through targeted advertising can limit the visibility of ads and display content only to e.g. a white or male target group within the context of housing – a scenario protected in the US under the Fair Housing Act as well as the Civil Rights Act. Another problematic example would be the more frequent display of an ad targeted to male instead of female or LGBTQ users within the context of hiring and recruiting. A scenario that could open the scope of the European anti-discrimination law like the equality of treatment in employment and occupation that specifically addresses gender and sexual orientation as characteristics protected by it.
Another important aspect is discrimination through proxies, which can be described as
a particularly pernicious subset of disparate impact. […I]t involves a facially neutral practice that disproportionately harms members of a protected class. But a practice producing a disparate impact only amounts to proxy discrimination when the usefulness to the discriminator of the facially neutral practice derives, at least in part, from the very fact that it produces a disparate impact.
Proxies can be in the form of user attributes like the ZIP code to exclude people of color, a specific race or humans talking a certain language that is used to exclude people from specific groups, audiences or offers.
Hence, machine learning (using skewed or biased data) can lead to the replica of real-world stereotypes and, through the use of opaque algorithmic decisions, can create new ones. This phenomenon is often referred to as algorithmic bias.
Another area in which discrimination may result from algorithmic bias is skewed training data within the process of the ad display. In such a case, the algorithm has found patterns and attributes in a data set that describe a certain kind of behavior or characteristic that is desired or preferred over others. An example might be that a system is evaluating historic data in the field of recruiting and comes to the conclusion that men are more qualified for financially well-compensated jobs than women because this pattern is represented within the data that was used to train the system on. This example illustrates the reciprocity between bias in the (human) behavior of the advertiser and the algorithmic functioning. To combat algorithm bias, many advocate for a complete ban to use targeting criteria like gender, age, race or ethnic origin within a certain context, a policy Google adopted 2019 for political content on its platform. However, even if targeting criteria are limited on platforms, targeted advertising can still be used in malicious ways by the right selection of proxies or e.g. other targeting criteria like Lookalike audiences. Therefore, regulation is needed to protect and equip democracy as well as the democratic process for a more digital future, to monitor the online sphere critically and even more strictly to prevent online manifestations of discrimination and prevent harm – online as well as offline.
Outlook of Targeted Advertising
We can conclude that targeted advertising is of growing importance, especially in scenarios that include digital spaces as the Metaverse or content that is otherwise more pervasive, otherwise new in form, shape or mean of presentation. The past years show that more users across social media platforms than ever before use platforms and other digital services that often are financed through ads. These firms therefore are generating their profit through the digital advertising process by setting a price for the user-attention of a particular audience in a specific point of time. The negative effects, as well as the positive effects like in the prevention of epidemics like COVID-19, that can be created through targeted advertising should be studied closely in order to provide platform specific solutions that not only repair discriminating architecture or decisions, but also find hidden human problems like bias in the system and actively steer against it. Furthermore, the consistent acting of platforms is important for tackling the problems described above.
The past years have brought a transition from the call for platform-self-regulation, which in the recent past has changed to the demand of governmental regulation, as expressed by the EU. Furthermore, researchers continue to argue for increased user transparency across platforms and a broader implementation of user information and empowerment in online services and choice.
And yet, only if more transparency is achieved and an understanding of the effects of targeted advertising on society is attained, will it be possible to improve design elements to prevent problematic usage of targeted advertising. Nevertheless, the user must become an informed actor in the process of ad targeting that can take informed decisions by her own and is aware of the degree to which their attention is being solicited. Finally, even if the above problems are solved, design patterns are widely accepted and many goals are achieved, some areas of targeting may however be completely still outlawed from use in the future.
The opinions expressed in this text are solely that of the author/s and do not necessarily reflect the views of IPPI and/or its partners.
Share this Post
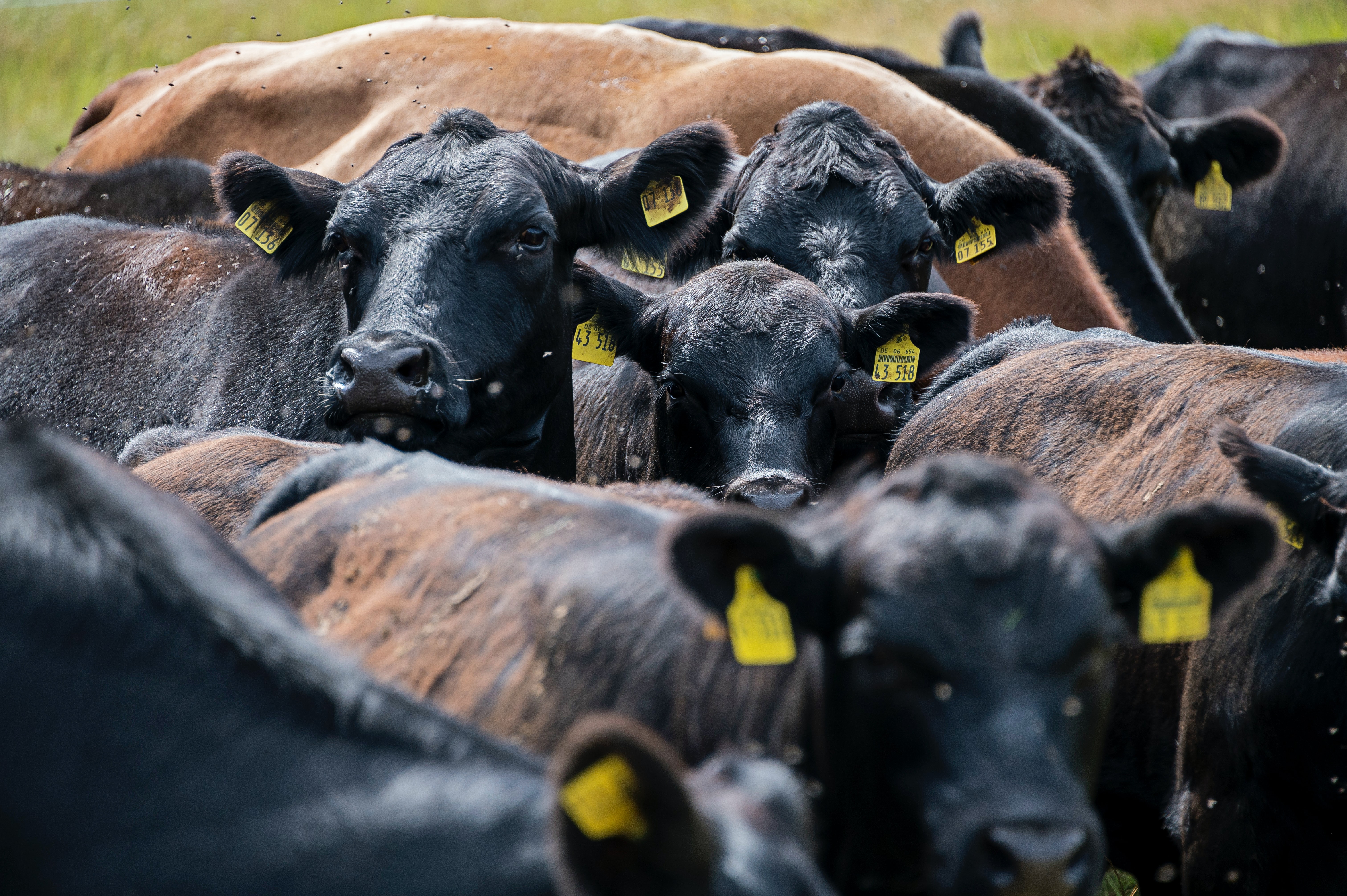
What is the relation between meat consumption and climate change?
Meat is big business. The global appetite for meat – which amounts to some 350 million tons every…
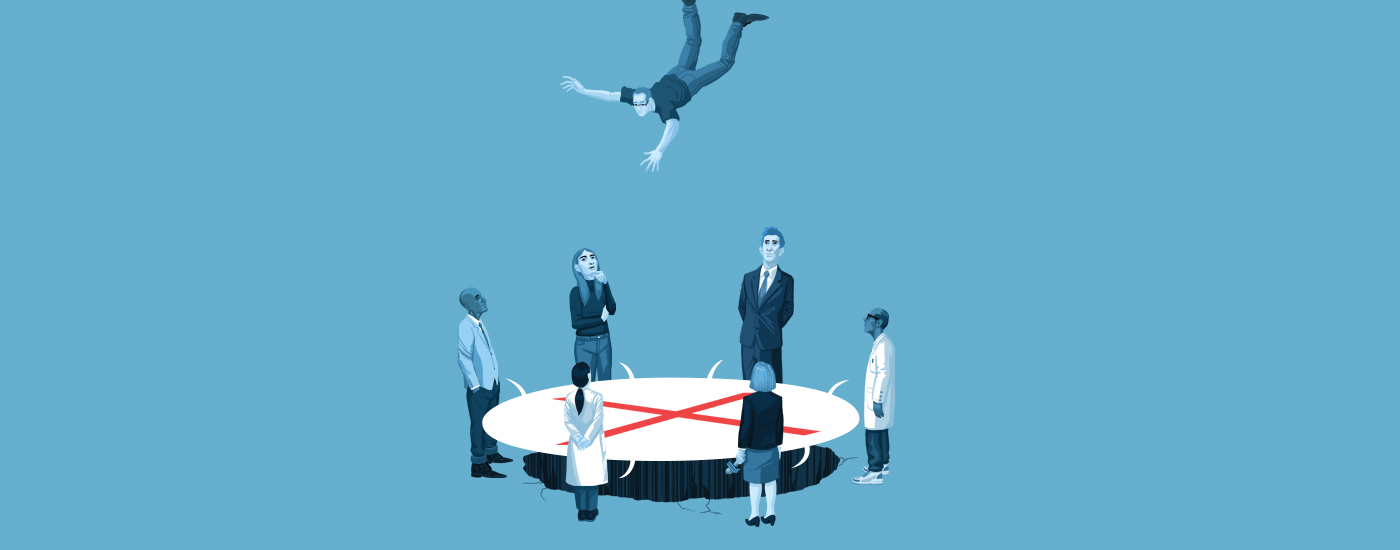
Trust, Democratic Resilience, and the Infodemic
Authors: Dr. Lena Frischlich and Dr. Edda Humprecht Trust and its Role in Combating Disinformation The world has…
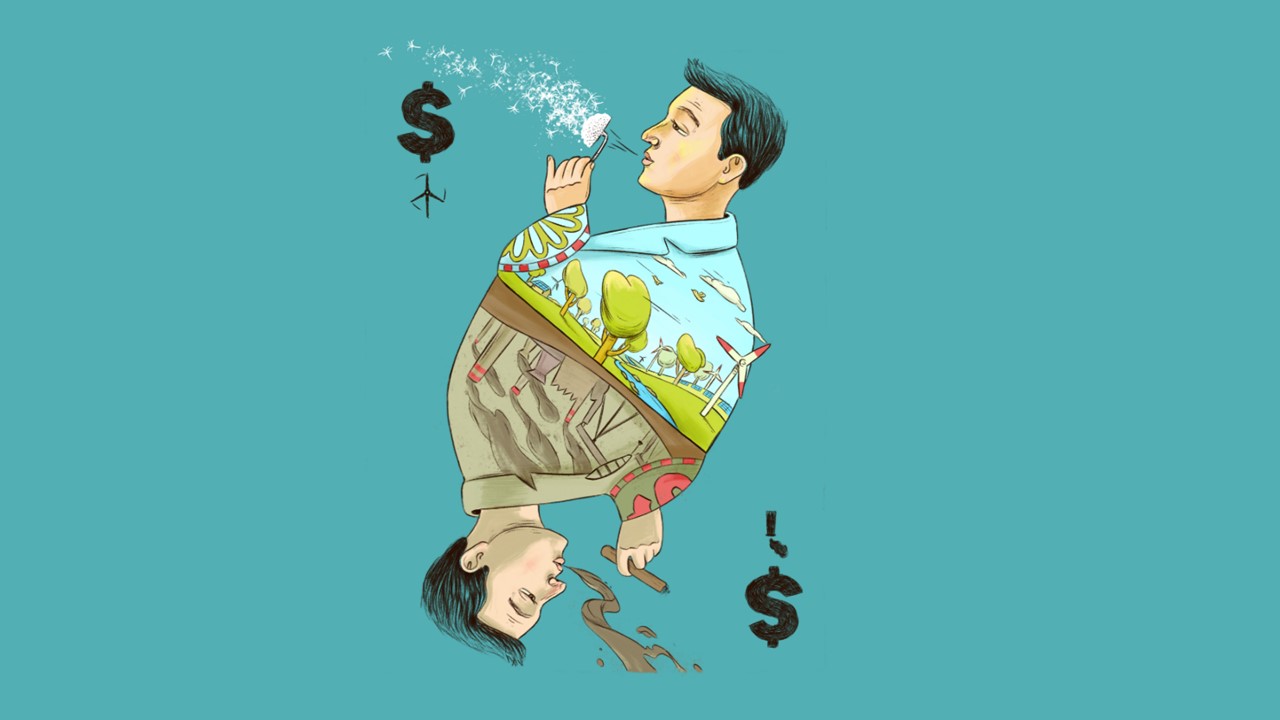
Bridging Environmental Frameworks Across Borders: Overview of the European Union’s Green Taxonomy and its Relevance beyond Europe
The European Union’s “Green Deal,” initiated by the 2019-elected EU Commission, stands as a symbol of Europe’s resolute…