Race against the Machine? The role of Technological Change for Employment, Wages and Inequality
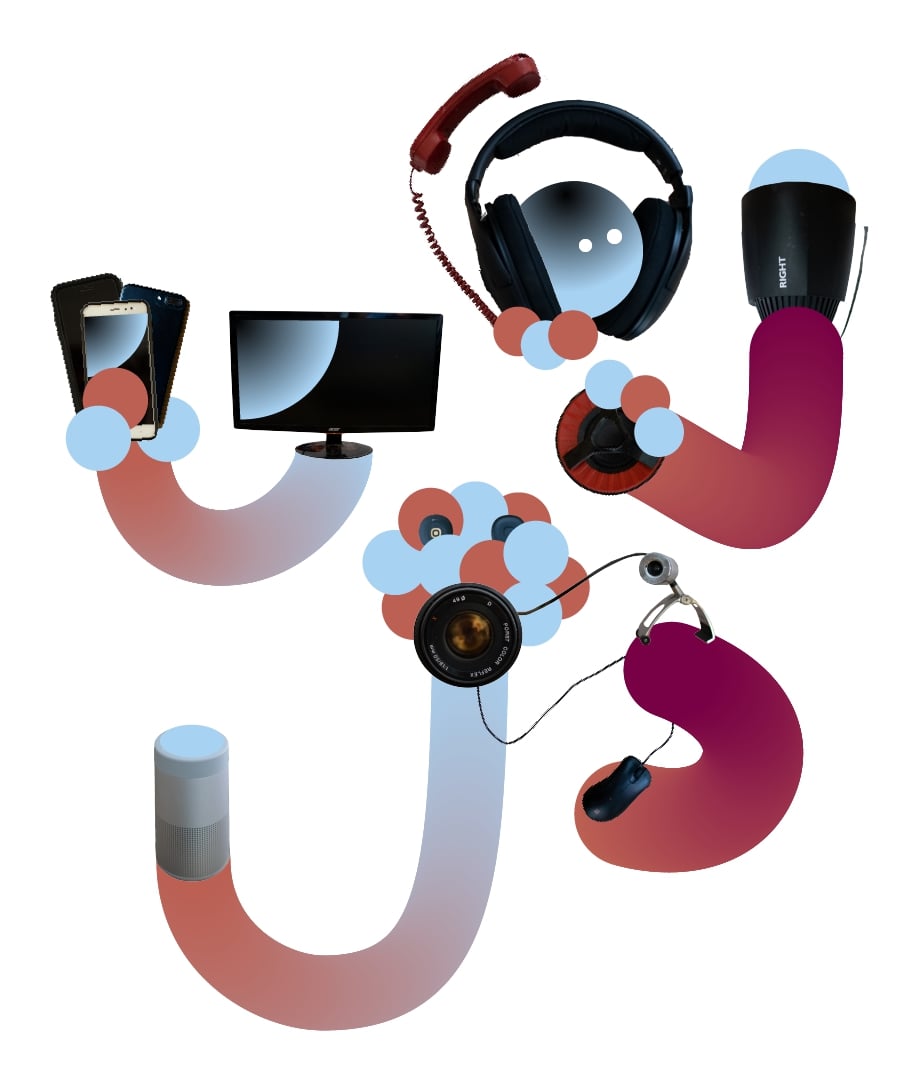
Share this Post
This Explainer provides interested non-experts with the current state of the scientific debate on the role of technological change on employment, wages and inequality. The aim is to help readers develop realistic expectations about the potential effects of digitization on the labor market, challenging the popular fears of a “jobless future” with scientific evidence.
Introduction
Computing power has increased at the enormously rapid rate of 45 percent per year since 1945 (Nordhaus 2007) and Machine Learning methods increasingly make it possible to automate tasks that previously seemed to be reserved for humans – even those of high-skilled workers (Brynjolfsson et al. 2019, Brynjolfsson and McAffee 2015, Pratt 2015). This has led to the widespread fear of a “jobless future” in the public debate, fueled by feasibility studies, which claim that about half of U.S. jobs are “at risk of computerization” (Frey and Osborne, 2017).
Are we about to face a world where robots take our jobs, creating mass unemployment? This article reviews the recent scientific evidence on the topic to discuss how new technologies might affect employment, wages, and inequality.
The “false dichotomy”
The current scientific debate builds on the concept of Routine Replacing Technological Change (RRTC), introduced by Autor et al. (2003). The underlying idea is that new computer-controlled technologies are particularly efficient at performing so-called routine tasks. Routine tasks follow well-defined rules that are easy to codify. This makes them suitable for automation through computer-controlled machines, a scenario in which computers replace humans in middle-wage jobs, leading to job polarization – that is, the hollowing out of middle-wage jobs with increasing shares of both low- and high-wage jobs. Autor et al. (2003) and Goos and Manning (2006) show that this indeed happened in the U.S., and Goos et al. (2009, 2014) document it for Europe.
Most of the scientific debate has focused on the consequences of technological change for skills and inequality, with only a little attention paid to the effect on number of jobs available. One of the main reasons has been that economic models predicted net employment losses only under unrealistic scenarios. In addition, there was little empirical evidence indicating that new technologies cause mass unemployment. Acemoglu and Restrepo (2018) called this the “false dichotomy” between the economic debate on the one hand, which largely overlooked the possibility of mass scale technology-induced unemployment, and the excessive fears of mass technological unemployment in the public debate on the other.
Are half of all jobs “at risk”?
The focus in the scientific debate shifted from technology-induced inequality to concern about job loss when Frey and Osborne (2017) claimed that about half of the U.S. workforce was “at risk” of automation, due to the widespread attention that this study received in the public debate. Two important aspects of this study require further attention. The first issue concerns its methodology; the second issue concerns its interpretation.
Exaggeration of automation potentials
Let us first focus on the method. Frey and Osborne (2017) asked experts whether or not the tasks of a selected list of occupations could be performed by new technologies. They then used the task structures of these occupations from an occupation-level database and trained a machine-learning model to extrapolate the expert evaluations from the selected list of occupations that the experts evaluated to all occupations. From the resulting model, which predicted which jobs were automatable, they then calculated how many employees currently work in automatable occupations.
The weak point in this method is that by using an occupation-level database of what people usually do in specified occupations, there is an implicit assumption that everybody in a given occupation performs exactly those tasks. However, it is well known from worker-level studies that workers’ tasks vary vastly within occupations – two workers with the same occupational title often do different things (Autor and Handel 2013).
How much does that matter? Arntz et al. (2017) show that automation potentials drastically decline when using data on tasks at the worker level. The reason is that workers specialize in hard-to-automate tasks even within seemingly automatable occupations. Using data at the occupation level thus exaggerates automation potentials, a finding that several other studies confirm.
Misinterpreting automation potentials
The second issue is interpretation, and it has far-reaching effects on outcome. Frey and Osborne (2017) leave the interpretation of their results relatively open: They argue that these jobs are “at risk” but do not further explain what “at risk” actually means. Many journalists have exploited this ambiguity to interpret these findings as predictive of massive job losses. However, the predictions by Frey and Osborne (2017) solely refer to whether experts think that the job could in principle be done by a machine. They therefore reflect a potential to automate a given occupation, but are is not informative about potential job losses or unemployment. The disparity between potential outcome and prediction is due to three main reasons (for more details, see the work by Arntz et al. (2020)):
Slow adoption of new technologies
Firstly, technological feasibility neither implies that something is actually about to be implemented, nor that it will be implemented anytime soon. In fact, most studies find that the time that it takes for new technologies to be widely adopted is typically very long: Thirty years after the introduction of electricity, 50% of U.S. plants still remained non-electrified. Computers required 25 years to receive their long-term plateau of 5% of residential equipment. Technological potentials require a long time to unfold, something that Arntz et al. (2020) discuss in more detail in an overview article.
Workers adapt by taking over new tasks
Secondly, if new technologies are actually adopted, they do not typically displace the workers immediately, nor do they displace them in all tasks. Instead, the vast majority of the change actually takes place due to changing task structures within occupations rather than displacement of entire occupations. For example, Spitz-Oener (2006) found that computers reduced demand for humans in routine tasks, although 99% of the decline in workers’ routine tasks can be explained by a reduction in routine tasks in occupations that were initially routine-intense, and only 1% by declining shares of routine occupations. In other words, automatable jobs hardly ever disappear. Instead, workers in automatable jobs take over non-automatable tasks.
Technologies also create new jobs
Finally, new technologies, at the same time that they eliminate old jobs, generate new positions, so that the net effect could in fact also be positive. In response to the lack of economic models that can explain net negative employment effects, Acemoglu and Restrepo (2018) developed a new seminal framework to study – among other things – the employment effects of technological change. Their framework shows that phases of technological unemployment can arise when the rate at which machines learn and take over tasks that previously only humans could do (automation) exceeds the rate at which humans learn new tasks that machines cannot yet do (reinstatement). Their study thus highlights that the net effect of technological change on the number of jobs is an empirical question.
Recent empirical evidence on the employment effects of technological change
With the shift in the focus of the debate to the question of the net employment effect of automation, recent scientific articles increasingly empirically analyze what technological change means for the number of jobs. For example, Gregory et al. (forthcoming) show that RRTC actually led to net positive employment effects in the European Union. While they do find strong displacement effects, they also find that the compensating effects of RRTC via economic growth and positive spillovers to other sectors with slow automation not only compensate for but exceed the initial displacement effects, explaining why the net effect is positive.
While they provide these findings for computer-controlled technologies more broadly, other authors zoom-in on industrial robots and provide heterogeneous results, depending on the country context. For example, Graetz and Michaels (2018) did not find that robots reduce hours worked in a sample of 17 OECD countries, except among low-skilled workers. Dauth et al. (2021) find net neutral effects of robots in Germany, where employment gains in service occupations compensate for employment losses in manufacturing occupations. Acemoglu and Restrepo (2019), on the contrary, find net negative employment effects of robots in the U.S. Overall, the existing evidence speaks in favor of somewhat negative employment effects in the U.S., whereas there is no evidence of net negative effects in Europe. Significantly, the indications from all of these results are a far cry from a “jobless future” or mass technological unemployment.
The Future of Work?
What does this imply for the future of work? One cannot predict the future, but a scenario of mass technological unemployment seems unlikely given the existing scientific evidence. Nevertheless, the scientific evidence clearly shows that the structures of jobs change. The main challenges are that specific skills become obsolete, workers have to re-train, particularly low-skilled workers face increasing troubles in finding well-paid jobs, and the transition from declining jobs to new jobs is often arduous, long, and results in lower wages for those who cannot occupationally upgrade (see Arntz et al. 2020). The question of the future of work is thus more about the structure of jobs and rising inequality than it is about the number of jobs. The exaggerated fears of a jobless future have distracted the debate from these actual challenges. The debate should focus more on how to ensure that workers gain the skills that they need to succeed in the labor market and to fight rising inequality.
Further Reading
Arntz, Melanie, Terry Gregory und Ulrich Zierahn (2020), “Digitization and the Future of Work: Macroeconomic Consequences,” in: Klaus F. Zimmermann, Handbook of Labor, Human Resources and Population Economics, Springer, Cham, https://doi.org/10.1007/978-3-319-57365-6_11-1
[1] This article builds on earlier work by Arntz et al. (2020).
————————————————————————————————————————————————–
This explainer is published in the framework of the European-Israeli Forum for Technology and Society, a joint initiative by the Israel Public Policy Institute (IPPI) and the Heinrich Böll Stiftung Tel Aviv.
The opinions expressed in this text are solely that of the author/s and do not necessarily reflect the views of the Israel Public Policy Institute (IPPI) and/or the Heinrich Böll Stiftung Tel Aviv.
Share this Post
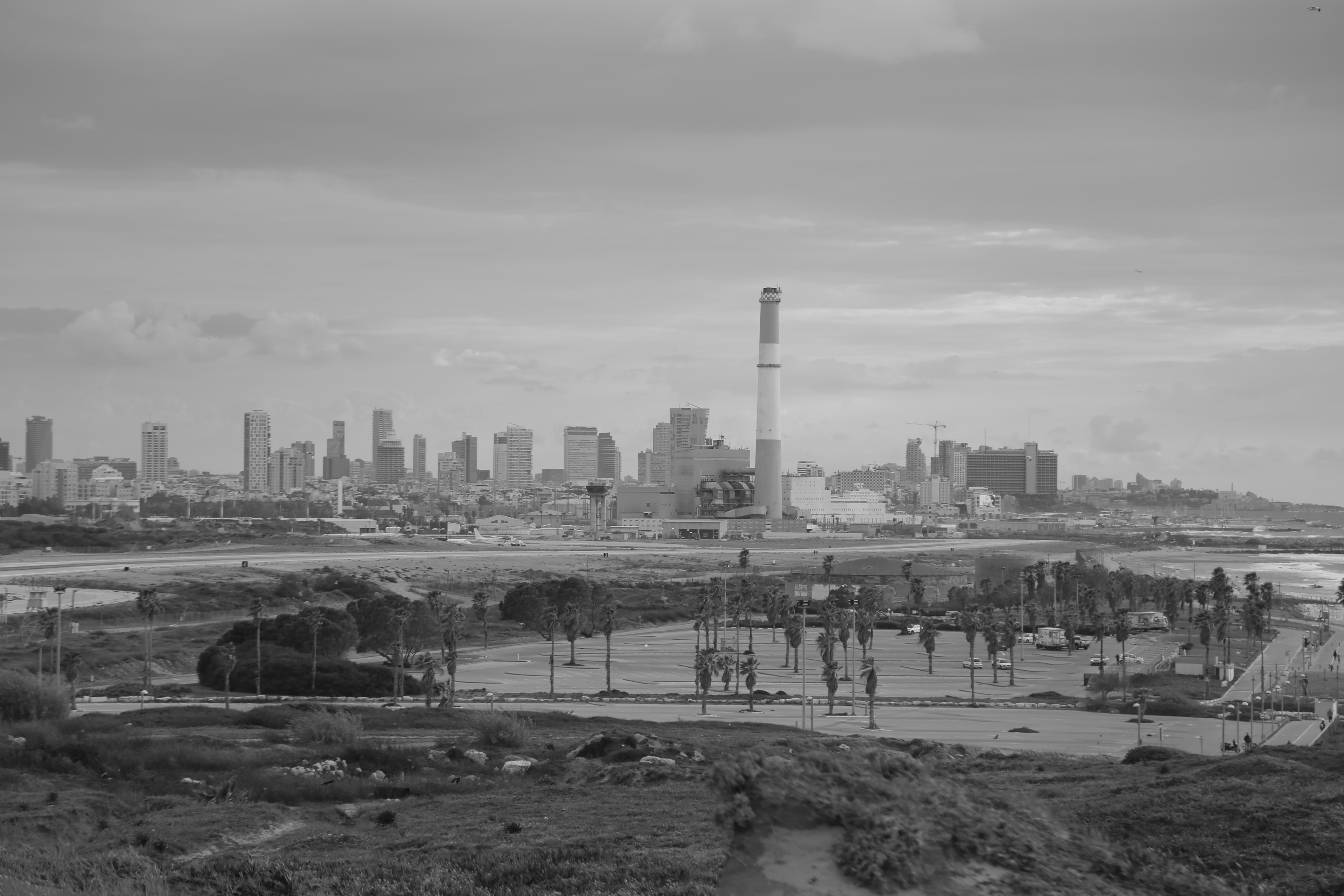
The Greening of Israeli Politics
The recent national elections in Israel in April 2019 saw an unprecedented rise in the presence of environmental…
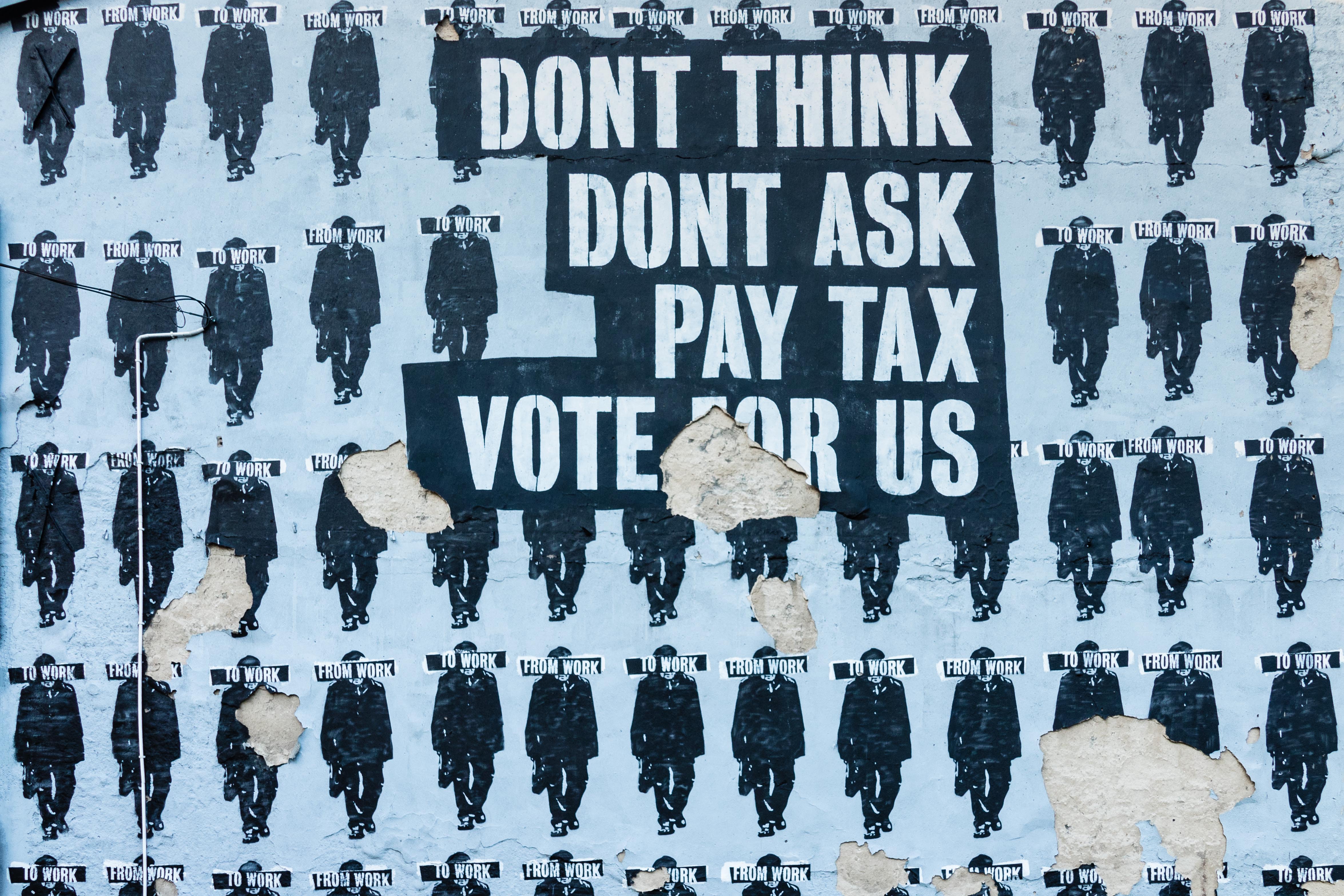
Updating Democracy for Future Generations: Adding a Fourth Branch to the Separation of Powers Model
Over the past two decades, political science has engaged in a lively debate about the “presentism” of democracies,…
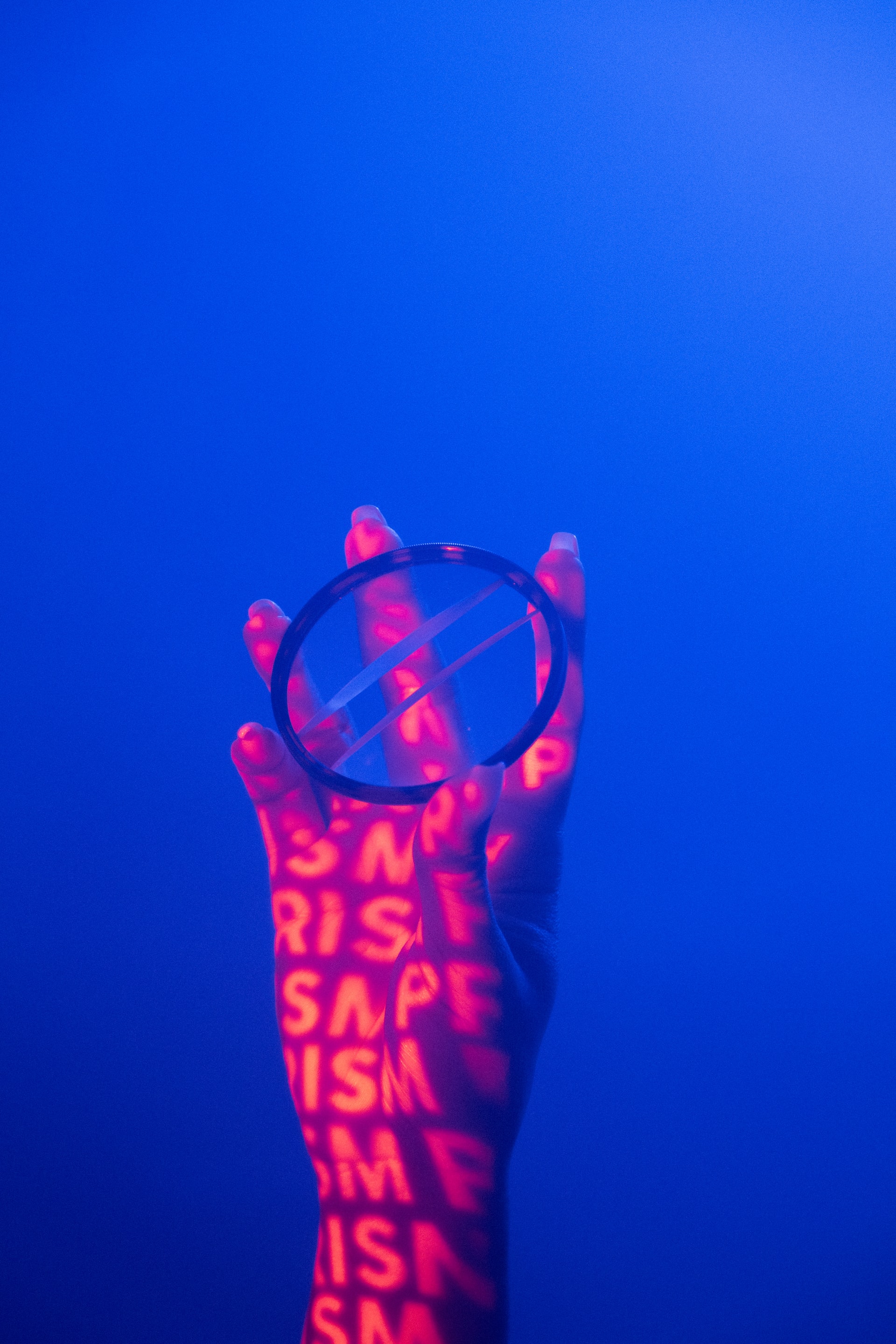
What are Filter Bubbles and Digital Echo Chambers?
Many experts are concerned that the curation of content on social media platforms limit our chances of encountering…