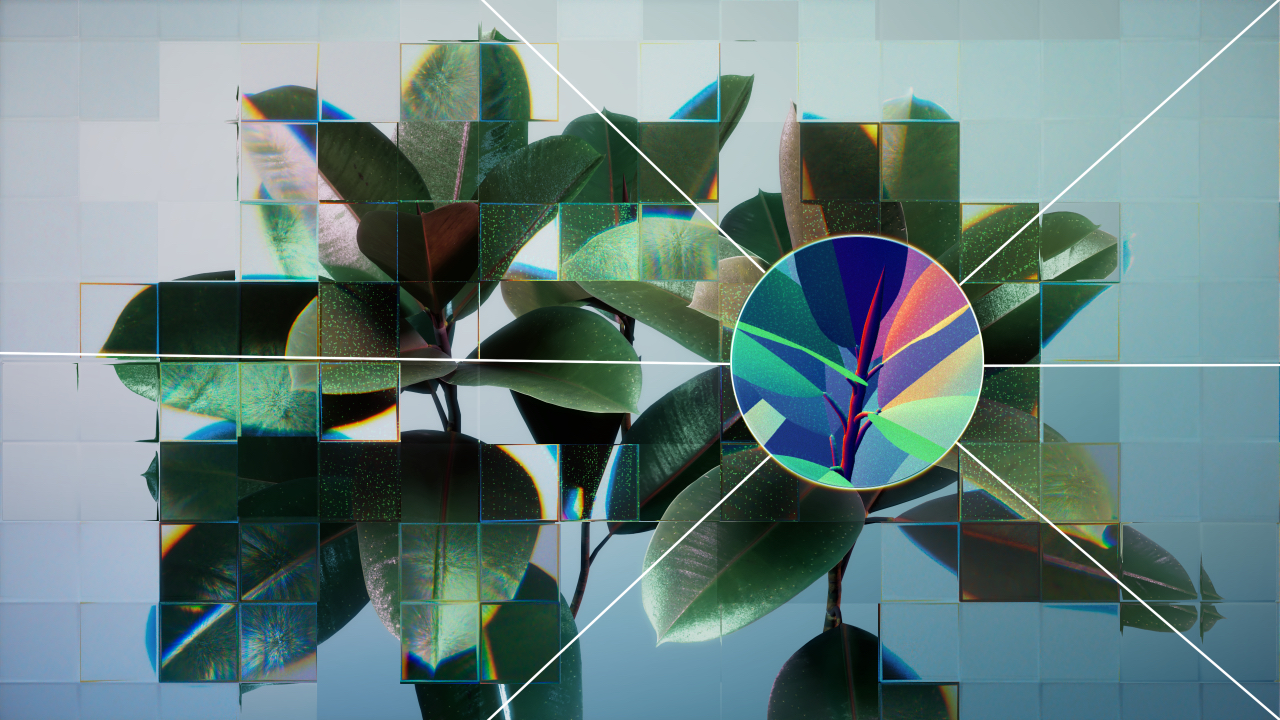
Share this Post
Authors: Eduardo Magrani & Francesca Portante d’Alessandro
How is artificial intelligence reshaping art authentication?
The image of the refined and articulate “art expert,” with thick frame glasses and a black turtleneck, is deeply embedded in the collective imaginary. However, could this figure (like many throughout history) become obsolete and replaced by an algorithm? Numerous startups and research projects have been exploring the possible applications of artificial intelligence within the art world, particularly for authentication purposes. Leading the charge is the Swiss startup Art Recognition, co-founded by Dr. Carina Popovici and Christiane Hoppe-Oehl, which utilizes neural networks and deep machine learning to authenticate artworks from a single photograph. A high quality image dataset of original paintings (often split into smaller patches to increase precision) is utilized to train the algorithm, which then identifies and matches specific characteristics in the analyzed work, ultimately submitting an authenticity report.
The challenges of traditional art authentication
Authenticity is a central issue in today’s art market. According to the estimation of Fine Art Expert Institute (FAEI) director Yann Walther, over 50% of works currently in circulation are fakes or misattributed. Taking into account the enormity of this figure, as well as the lofty prices art can reach at auctions, it is unsurprising that determining authenticity constitutes an essential precondition for sales and acquisitions to take place. Authentication carries even more weight when we consider that the circulation of fakes can even substantially alter cultural capital, art history, as well as affect the market price of artworks. Given the high stakes of this key process, which is complex and not without its flaws and fallacies, the possible uses and attendant advantages of AI in this field are exciting and numerous. The extent, however, to which an algorithm is actually able to outperform and replace traditional authentication processes is questionable, and will be the focus of this article.
Fine art authentication has traditionally relied on the combination of scientific analysis, connoisseurship and provenance, which all raise distinct issues. Scientific methods such as carbon dating, pigment analysis and x-rays can be used to add a layer of objectivity, yet are not infallible, as many forgers utilize original canvases and material from the forged period. Provenance, namely the information regarding ownership (comparable to the legal chain of custody), is also used to support authenticity claims. Sale auction catalogues, artists’ writings or letters, inventories and photographs can prove essential, but these can also be falsified; further, they are sometimes unavailable for older artworks.
Due to the ability of forgers to bypass both scientific analyses and provenance inquiries, connoisseurship is to this day the primary method of authentication, relying on an individual’s or association’s knowledge of an artist’s oeuvre and technique through careful observation. Despite its prevalence, however, like the realms of scientific method and provenance, it comes with its own liabilities. The major point of contention is the disproportionate power that individual experts and associations hold, which may easily lead to abuse and fraudulent behavior. This is exemplified in Simon-Whelan v. The Warhol Art Foundation, where the plaintiff accused the Andy Warhol Foundation of intentionally rejecting authentic works in order to inflate market prices by inducing scarcity. The risk of bias is particularly heightened in the case of periods and artists for which few experts are available. The problem posed by variations in expertise and methodology of each individual connoisseur has also raised significant criticism. While some experts are indeed academics and art historians, many artists’ foundations are composed of collectors and relatives who do not necessarily possess formal knowledge and expertise. Furthermore, the process of authentication in itself is often murky, whereby decisions are described only as an individual intuition or impression, with little (if any) explanation provided. Finally, perhaps the most relevant claim is that human intellect is indeed fallible, leading to misattribution.
Deep learning in art: Risks and biases
Within this context, the advantages of an AI system such as Art Recognition are clear, as it provides an unbiased, objective and independent algorithm. Firstly it expedites the entire process in practical terms, by obviating the need for shipping and deliberation times alongside insurance costs and other risks related to the transfer of potentially valuable artworks. The system only requires a high-quality image of the work, for which, according to the company website, an iPhone image sufficient. Furthermore, unlike many associations or authentication boards, it provides clients a detailed report of the decision, highlighting problematic areas in the artwork through the use of heat maps.
It is the minimization of risks of bias and fraud, however, that has been heralded as the most significant advantage of AI, as decisions are based on an impartial data-driven algorithm rather than on individuals’ discretionary judgement. Yet while the risks attending to individual judgement are certainly greatly reduced using AI, they cannot be removed completely. The algorithm is in fact designed to replicate an expert’s formalist analysis and possibly improve on it; it does not adopt a new and revolutionary approach. Arguably, then, it could be misled just as easily as a human expert by well executed copies, especially if no physical scientific analysis is carried out simultaneously. This risk is further amplified by the possibility that fakes could be present in the training data set, thus influencing the outcome. While Art Recognition ensures the reliability of the training data set (which includes only catalogue raisonné and museum works), it should be noted that numerous academic critics have cited the dubious authenticity of many high-profile works in several museum collections and catalogues raisonnés. The use of photographic and digitally scaled reproductions may also present an obstacle, introducing distortions, shadows and altering pigments, which may affect the algorithm’s decision. Finally, by focusing on artists’ visual compositional elements (and brushstrokes specifically), the algorithm may be inherently biased towards certain styles and artists. While this formalist approach would suit figurative styles such as impressionism (whose main characteristic is visible broad brushstrokes), would it be as accurate with abstract works or sculpture? Similarly, as the algorithm is trained through an image data set, would the size of an artists’ oeuvre influence the outcome? Art Recognition claims that for an accurate result, at least 200 original works need to be present in the training set, with more complex artists requiring a higher number in order to fully capture style variations. This seems to confirm the concern that the algorithm would more accurately analyze prolific painters (like Picasso), compared to artists who only have a handful of works. Finally, would the algorithm truly be independent? While the algorithm independently deliberates on the authenticity claim, it does so based on the training data set that it is provided by the company. Would it therefore still be possible for individuals to control the process for their gain, by deliberately introducing fakes and manipulating the training set? Would those who control the algorithm control the whole process? Taking into account such issues, it is clear that AI (at least currently) is not able to completely replace traditional methods of analysis, but rather may provide additional guidance for experts to take into account when deliberating.
Would AI revolutionize the authentication process?
The use of AI authentication, while potentially adding a layer of objectivity to the authentication process, potentially raises legal issues in regards to liability. This is because the AI decision does not exist in an informational vacuum, but rather has costly and significant real-art-world implications. The process of authentication has historically given rise to substantial litigation, leading scholars to refrain from expressing opinions and impelling foundations to disband in order to avoid liability. The Andy Warhol Foundation, for example, famously dissolved due to unsustainable litigation costs (around 10 million dollars over 10 lawsuits in 15 years). Action is taken not only when authenticated works are later exposed as forgeries, but more often when experts refuse to authenticate works despite abundant compelling evidence.
Since the algorithm is not completely infallible and accurate, as established above, Art Recognition’s warranty and contract exclude any liability resulting from an incorrect AI Report. This places even greater pressure on authentication boards and experts who are responsible for the final decision regarding authenticity. Could an individual sue an expert who erroneously relied on an incorrect AI report? A further novel potential scenario is liability charges against experts who choose to completely disregard AI reports. In order to avoid litigation, experts would then not only tend to be more conservative in their decisions, but would also be less likely to disagree with the algorithm’s decision. This is problematic as it would make the use of experts superfluous, despite the position even of the founders of Art Recognition that the system should be used as supporting evidence and not as the ultimate arbiter.
It is clear that current AI technology, though useful in addressing several problems relating to connoisseurship, is by no means incontestable or at a stage where it can fully supplant traditional methods of authentication. The limitations of algorithms and training sets should be taken into consideration, resulting in use of AI technology in conjunction with other methodologies, thus allowing for peer review and preventing a single person or system from exercising all discretion in attribution matters. The art world’s general skepticism towards innovation, alongside the pervasive suspicions relating to algorithms’ ability to detect forgeries, ensures a limited use of such technology by institutions and collectors, at least for the moment. Once this technology does become pervasive as it continues to develop and enter the mainstream, one can expect interesting litigation, possibly expanding liability and duty-of-care regimes in the art market (an area which is arguably currently underdeveloped).
Further readings
https://art-recognition.com/about-us/
“Over 50 Percent of Art is Fake,” Artnet News, (October 13, 2014) https://news.artnet.com/market/over-50-percent-of-art-is-fake-130821
Simon-Whelan v. The Andy Warhol Foundation for the Visual Arts, Inc., No. 07 Civ. 6423 (LTS) (Southern District of New York. May 26, 2009)
https://art-recognition.com/faq/
Daniel Grant, “New Legislation Would Protect Art Authenticators Against ‘Nuisance’ Lawsuits.” The New York Observer, (June 4, 2014)https://observer.com/2014/06/dont-shoot-the-messenger-if-passed-new-legislation-would-protect-art-authenticators-against-nuisance-lawsuits/
The opinions expressed in this text are solely that of the author/s and do not necessarily reflect the views of IPPI and/or its partners.
Share this Post
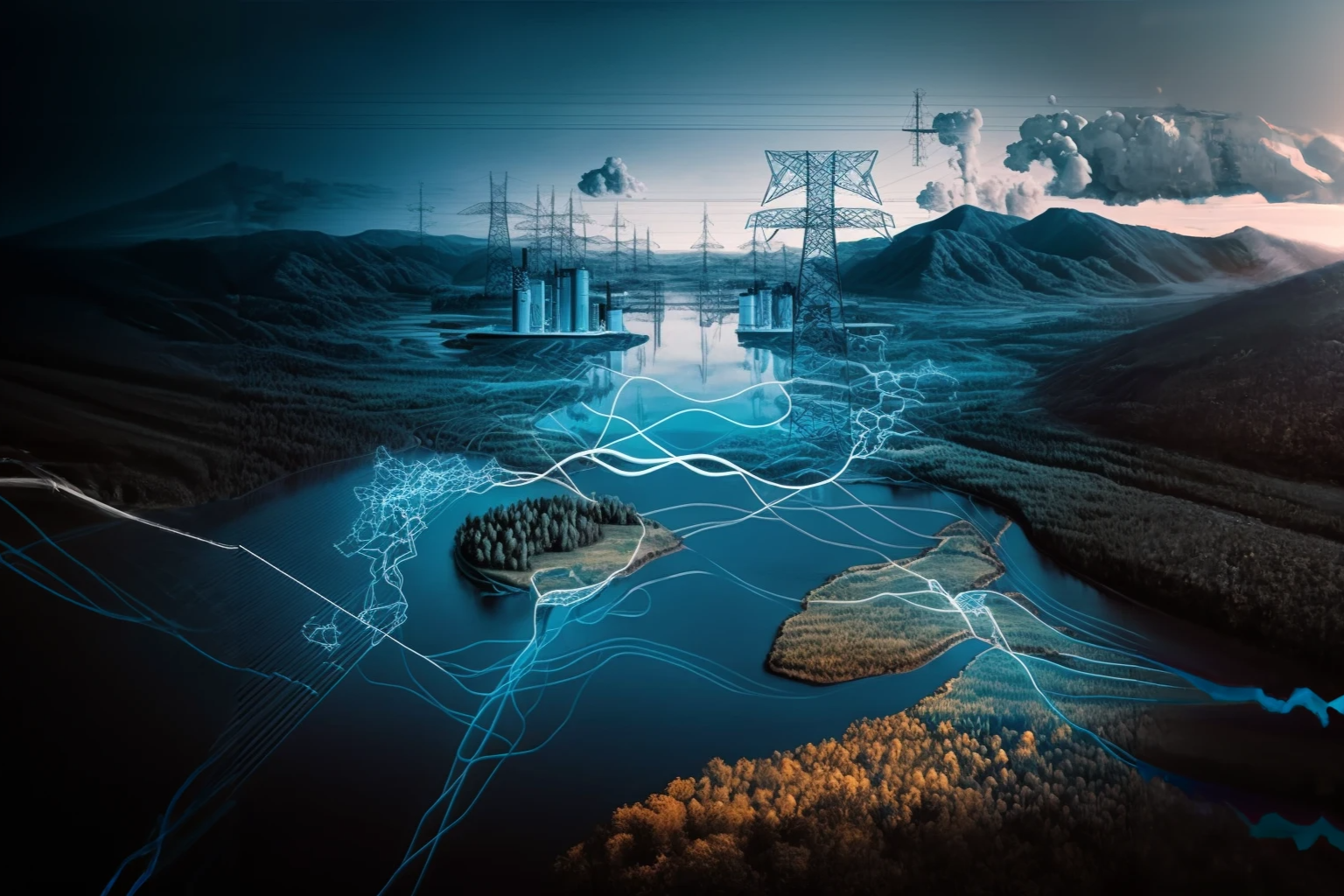
Power-to-X – What is it and how does it work?
The world is undergoing a transition towards renewable energy sources to combat climate change. One of the main…
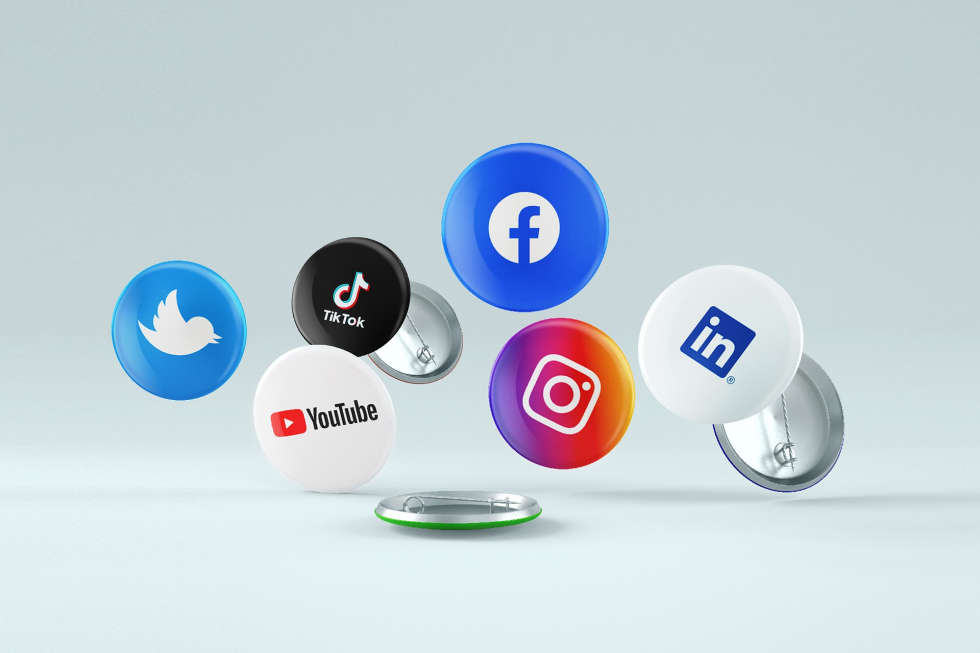
"The basis for democratic discourse in the age of social media is skewed"
Disinfo Talks is an interview series with experts that tackle the challenge of disinformation through different prisms. Our talks…
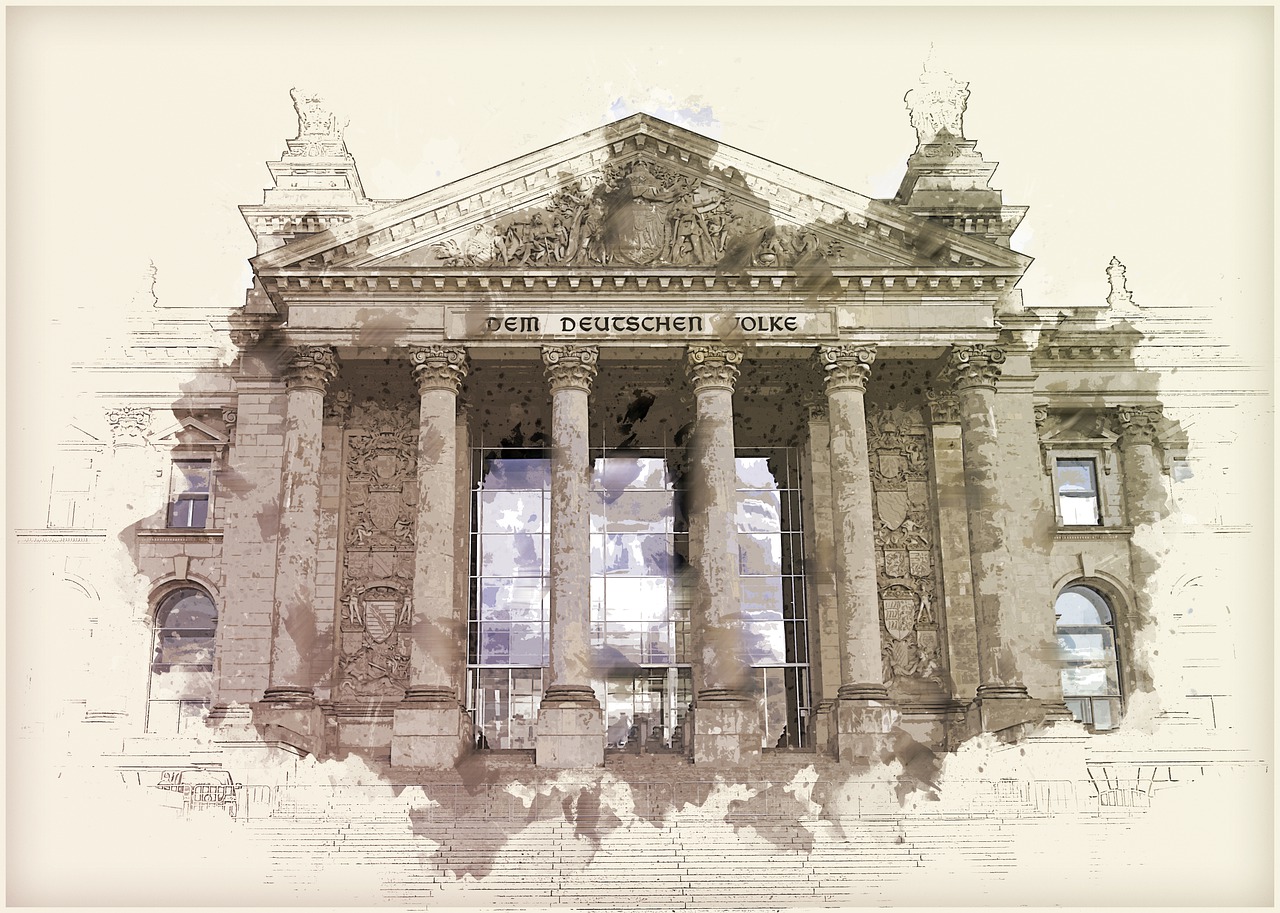
Containing authoritarianism in the digital age: Platform governance in view of Germany’s constitutional order
Authors: Martin Fertmann & Jan Rau Current Challenges in Governing Digital Spaces Terror attacks in Christchurch and Halle…